[toc]html
Pandas 是基於NumPy 的一種工具,該工具是爲了解決數據分析任務而建立的。 Pandas歸入了大量庫和一些標準的數據模型,提供了大量能使咱們快速便捷地處理數據的函數和方法。 主要包含兩種數據類型:Series和DataFramemysql
相關幫助文檔sql
示例代碼以下數據庫
from sqlalchemy import create_engine import pandas as pd username = '用戶名' password = '密碼' host = '鏈接地址' db = '數據庫' port = 端口號 link = f'''mysql+pymysql://{username}:{password}@{host}:{port}/{db}?charset=utf8''' engine = create_engine(link, pool_recycle=3600)
核心方法read_sql數組
log:pd.DataFrame = pd.read_sql("SELECT * FROM log ORDER BY id DESC ",engine)
執行結果以下 app
import datetime log[log['create_time'] > '2020-01-15 16:14:22']
logs[['user_id','type']]
logs['type']
如今我須要將user_id對應的用戶名找出來,示例代碼以下dom
#查詢出全部的用戶,以便將log和users作join users:pd.DataFrame=pd.read_sql("SELECT * FROM users",engine) users
* users和log的字段太多,先作一下篩選函數
log=log[['type','user_id','project_id','create_time']] users=users[['id','username','real_name']]
執行join,使用merge方法,how指定左連,left_on指定左表使用的字段, right_on指定右表使用的字段工具
log.merge(users,how='left',left_on='user_id',right_on='id')
drop方法,axis爲0表明行,1表明列spa
renameRes.drop('建立時間',axis=1)
dropRes.groupby(['type','real_name']).count()
groupby也能夠能夠傳入一個可以訪問索引上字段的函數
rng=pd.date_range('1/1/2020',periods=100,freq='D') ts=pd.Series(np.random.randn(len(rng)),index=rng) ts.groupby(lambda x:x.month).mean() 2020-01-31 0.182420 2020-02-29 0.200134 2020-03-31 -0.108818 2020-04-30 -0.187426 Freq: M, dtype: float64
by指定字段,ascending指定升序仍是降序
log.sort_values(by='user_id',ascending=False)
默認groupby後的結果是行索引是groupby的字段
log.merge(users,how='left',left_on='user_id',right_on='id').groupby('type').count()
groupby指定參數as_index
log.merge(users,how='left',left_on='user_id',right_on='id').groupby('type',as_index=False).count()
另外,還能夠count完後直接調用reset_index方法
log.merge(users,how='left',left_on='user_id',right_on='id').groupby('type').count().reset_index()
log.T
使用rename方法,傳遞一個字典便可,以下
pd.DataFrame = res[['type','username','real_name','create_time']].rename({'create_time':'建立時間'},axis=1)
log['create_time'].astype(str)
count是必須依賴其餘列作統計的,當只有一列的時候如何還使用count,是看不出統計字段的,正確的方法應該是使用size
test4=pd.read_sql("SELECT `type` FROM log LIMIT 100",engine) test4.groupby('type').size()
例如,要將create_time轉爲YY-MM-DD格式,可使用函數.dt.date
log['create_time'].dt.date
具體方法能夠參考Series的API文檔的Datetime操做
例如,轉爲大寫
log['type'].str.upper()
具體方法能夠參考Series的API文檔的字符串操做
簡單的理解就是一個更高級的groupby功能
df = pd.DataFrame({'foo': ['one', 'one', 'one', 'two', 'two', 'two'], 'bar': ['A', 'B', 'C', 'A', 'B', 'C'], 'baz': [1, 2, 3, 4, 5, 6], 'zoo': ['x', 'y', 'z', 'q', 'w', 't']}) df.pivot(index='foo', columns='bar', values='baz')
pivot_table支持分組後再聚合操做
df = pd.DataFrame({"A": ["foo", "foo", "foo", "foo", "foo", "bar", "bar", "bar", "bar"], "B": ["one", "one", "one", "two", "two", "one", "one", "two", "two"], "C": ["small", "large", "large", "small", "small", "large", "small", "small", "large"], "D": [1, 2, 2, 3, 3, 4, 5, 6, 7], "E": [2, 4, 5, 5, 6, 6, 8, 9, 9]} )
根據ABC分組,計算D的值,AB爲行索引,C爲列索引再使用sum函數,以下
df.pivot_table(values='D', index=['A', 'B'], columns=['C'], aggfunc=np.sum, fill_value=0)
通常使用matplotlib進行繪圖 例如,統計全部的操做日誌最多的前10個繪製直方圖 先取出這些數據,以下
#獲取全部操做類型最多的10條數據 countRes=log.groupby('type',as_index=False).count().drop(['create_time','project_id'],axis=1).rename({'user_id':'count'},axis=1).sort_values(by='count',ascending=False).head(10)
爲了讓圖是遞增的狀態,咱們反轉一下
countRes=countRes.iloc[::-1]
再使用matplotlib繪製直方圖
import matplotlib.pyplot as plt plt.barh(countRes['type'],countRes['count'])
apply(DataFrame) DataFrame的函數,apply將函數應用到由各列或行所造成的一維數組上,默認是傳入列的Series,能夠指定axis=1傳入行
applymap(DataFrame) DataFrame的函數,對每一個元素應用函數
map/apply(Series) 對應Dataframe的applymap
pands使用NaN(Not a Number)表示缺失數據,處理方法有
data = pd.Series(np.random.randn(10), index=[ ['a','a','a','b','b','b','c','c','d','d'], [1, 2, 3, 1, 2, 3, 1, 2, 2, 3] ] )
選取一個子集
data['b']
選取多個子集
data['b':'c']
選取內層:,號後面添加行號
data[:,2]
#Series轉換爲DataFrame data.unstack()
#將DataFrame轉換爲一個Series data.unstack().stack()
frame=pd.DataFrame(np.arange(12).reshape((4,3)), index=[ ['a','a','b','b'], [1,2,1,2] ], columns=[ ['Ohio','Ohio','Colorado'], ['Green','Red','Green'] ], )
frame.index.names=['key1','key2'] frame.columns.names=['state','color']
frame.swaplevel('key1','key2')
frame.sort_index(level=0,ascending=False)
df=pd.DataFrame({ 'a':range(7), 'b':range(7,0,-1), 'c':['one','one','one','two','two','two','two'], 'd':[0,1,2,0,1,2,3] }) df.set_index(['c','d'])
data=pd.DataFrame({ 'k1':['one']*3 + ['two']*4, 'kw':[1,1,2,3,3,4,4] })
data.drop_duplicates()
df = pd.DataFrame({'foo': ['one', 'one', 'one', 'two', 'two', 'two'], 'bar': ['A', 'B', 'C', 'A', 'B', 'C'], 'baz': [1, 2, 3, 4, 5, 6], 'zoo': ['x', 'y', 'z', 'q', 'w', 't']}) ```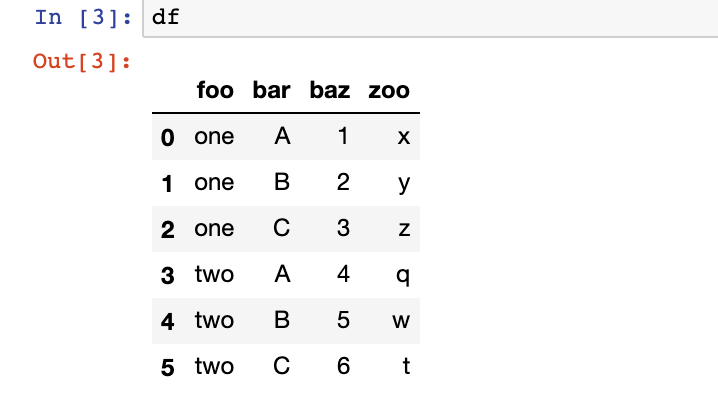 替換A和B爲chenqionghe,第一個參數爲查找值,第二個參數爲替換的值
df.replace(['A','B'],'chenqionghe')
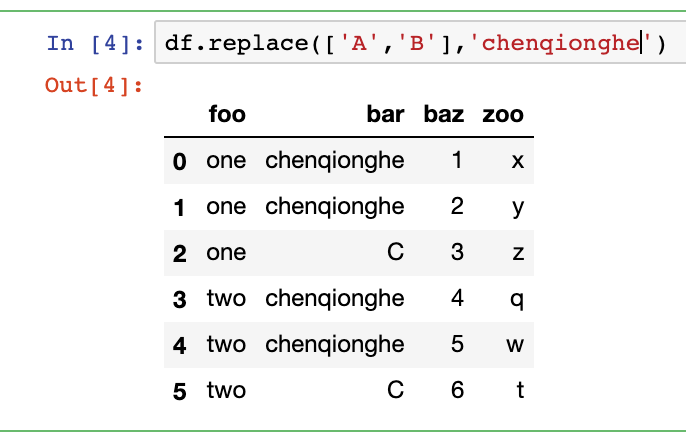 也能夠傳入字典,替換多個值
df.replace({'A':'cqh','B':'chenqionghe'})
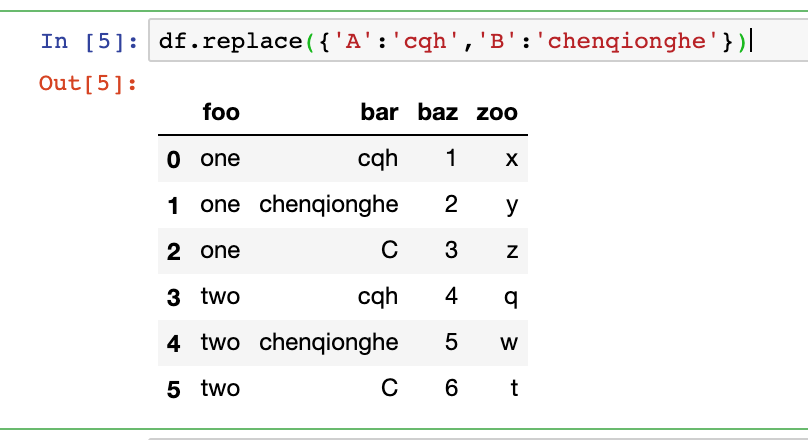 # 二11、如何鏈接兩個dataframe-concat
df1=DataFrame(np.arange(6).reshape(3,2), index=list('abc'), columns=['one','two'] ) df2=DataFrame(5+np.arange(4).reshape(2,2), index=list('ac'), columns=['three','four'] )
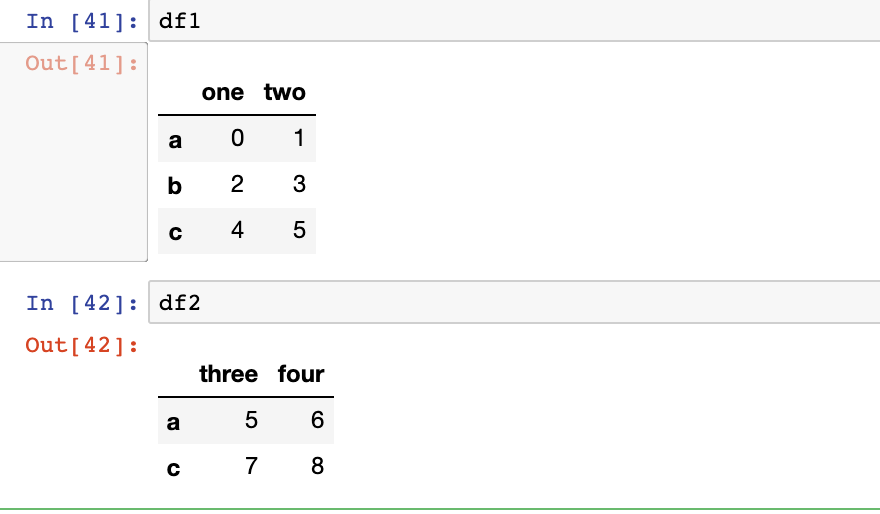 列拼接
pd.concat([df1,df2],sort=False)
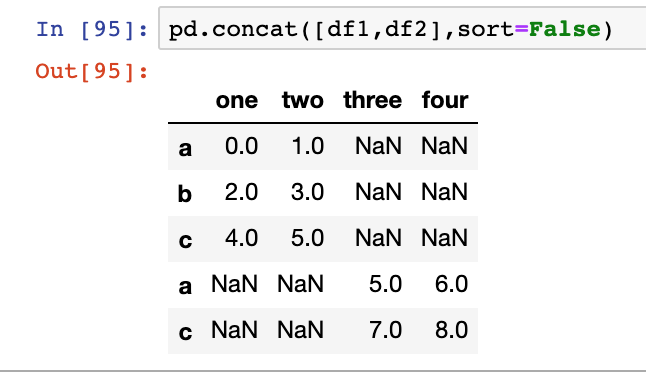 行拼接
pd.concat([df1,df2],axis=1,sort=False)
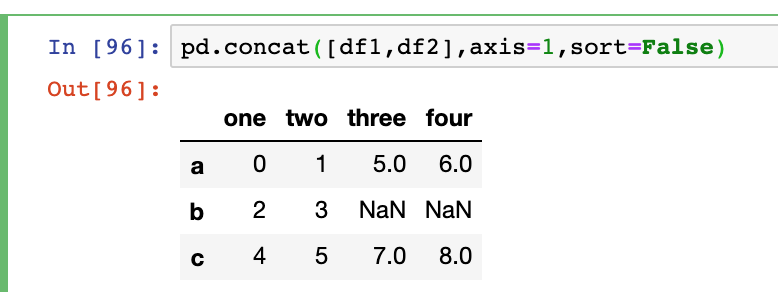 # 二12、如何從新設置索引-reindex
frame=pd.DataFrame(np.arange(9).reshape((3,3)), index=['a','b','c'], columns=['light','weight','baby'] )
#默認修改行索引 frame2=frame.reindex(['a','b','c','d'])
#同時修改行索引和列索引 frame.reindex(index=['a','b','c','d'],columns=['light','gym','muscle'])
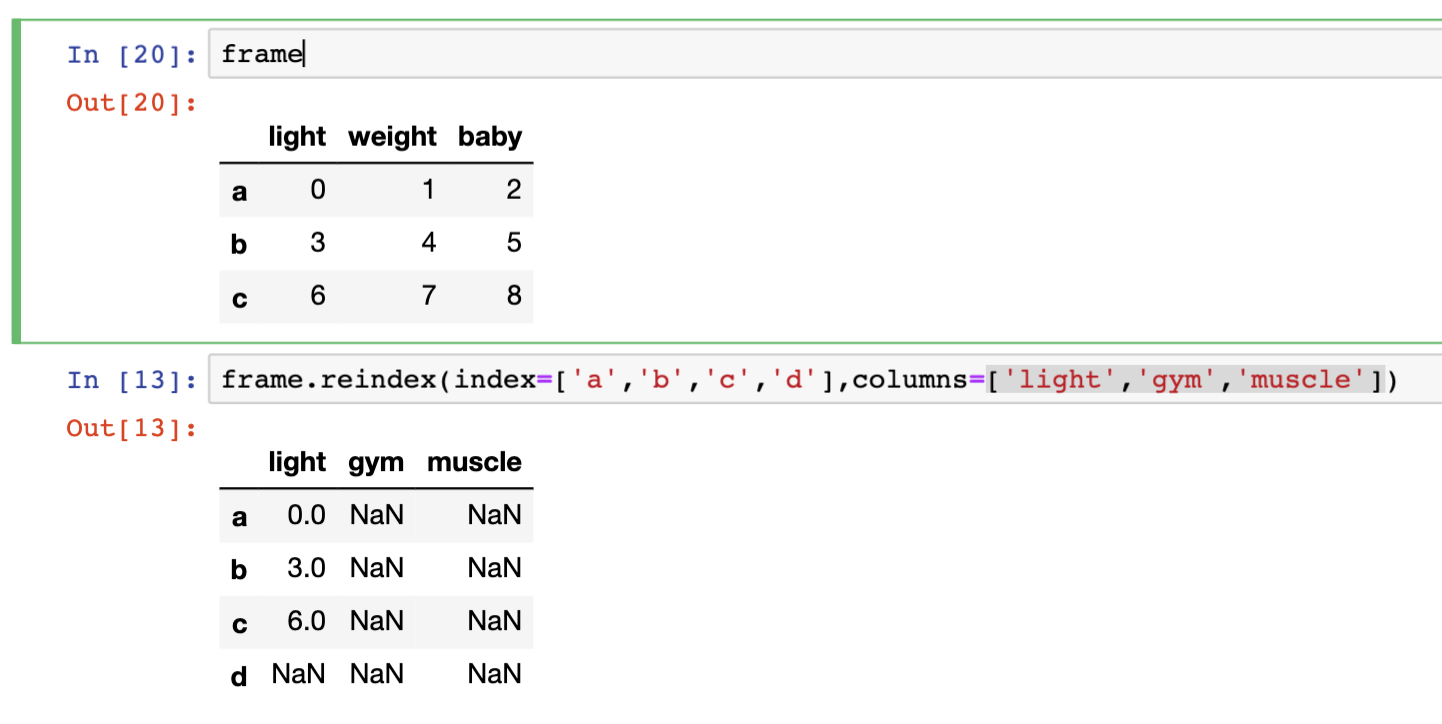 # 二12、如何從新採樣-resample 建立3個週三的時間序列
ts1=pd.Series( np.random.randn(3), index=pd.date_range('2020-6-13',periods=3,freq='W-WED') )
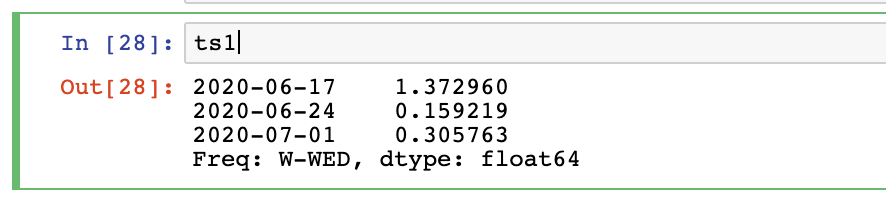 升採樣轉爲每一個工做日
ts1.resample('B').asfreq()
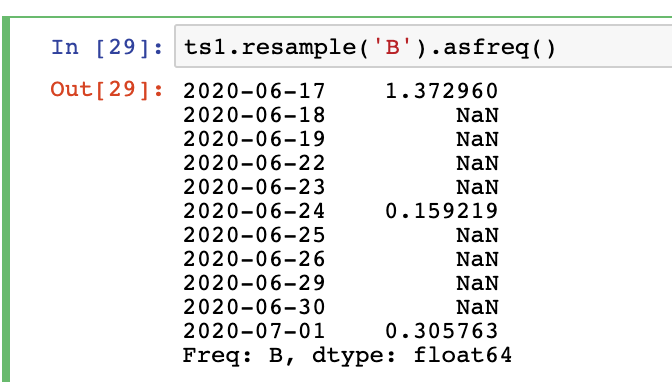 指定爲ffill的填充
ts1.resample('B').ffill()
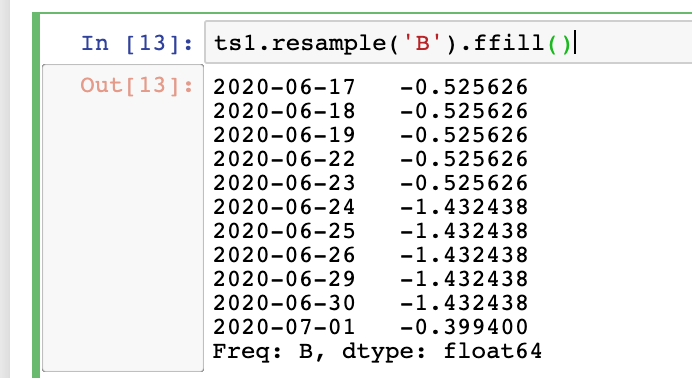 # 二十3、如何打補丁-combine_first combine_first至關於用參數對象中的數據爲調用者對象的缺失數據」打補丁「
df1=pd.DataFrame({ 'a':[1,np.nan,5,np.nan], 'b':[np.nan,2,np.nan,6], 'c':range(2,18,4) }) df2=pd.DataFrame({ 'a':[5,4,np.nan,3,7], 'b':['chenqionghe',3,4,6,8] })
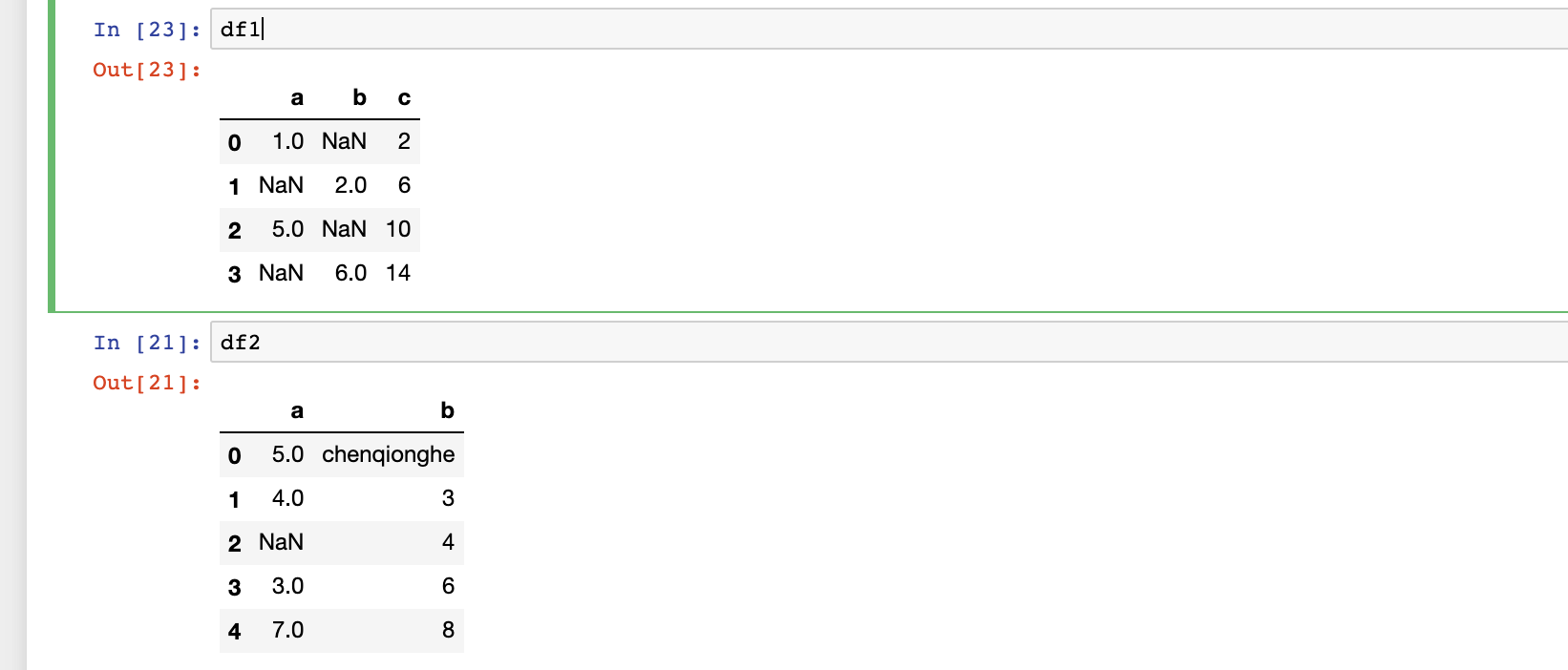 而後咱們能夠用df2的值給d1打補丁,以下
df1.combine_first(df2)
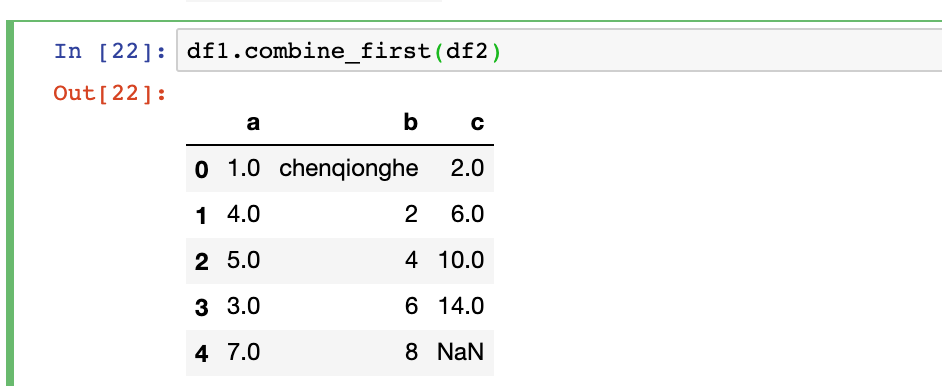 # 二十4、如何進行排名-rank
a=pd.DataFrame(np.arange(60).reshape(10,6),columns=['a','b','c','d','e','f'])
默認是對行進行排序,以下
a.rank()
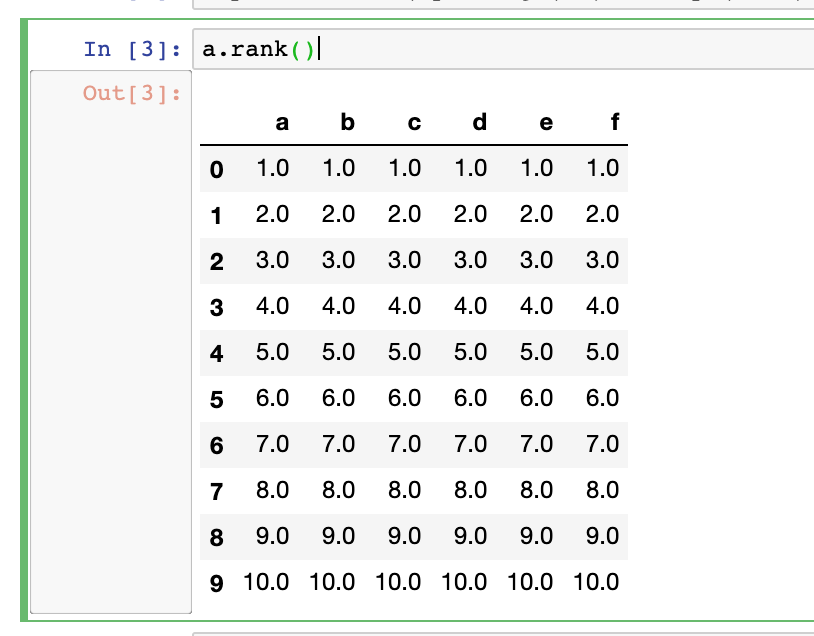 能夠傳axis=1對列進行排序
a.rank(axis=1)
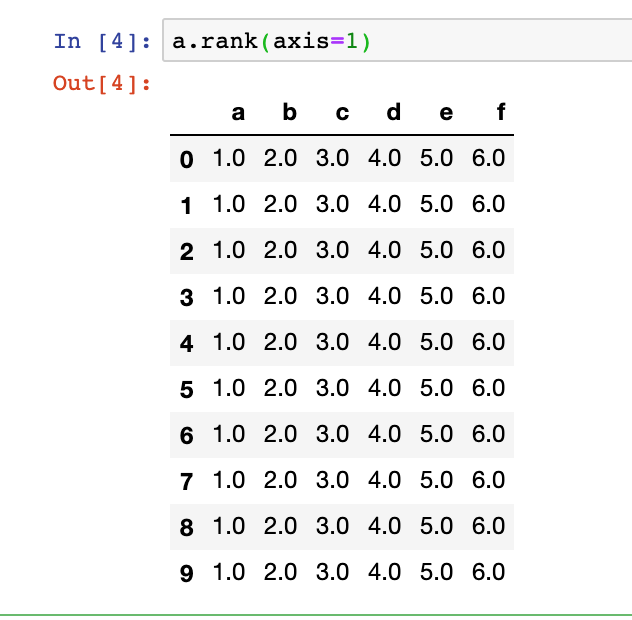 默認是升序,能夠傳入ascending=False進行降序
a.rank(ascending=False)
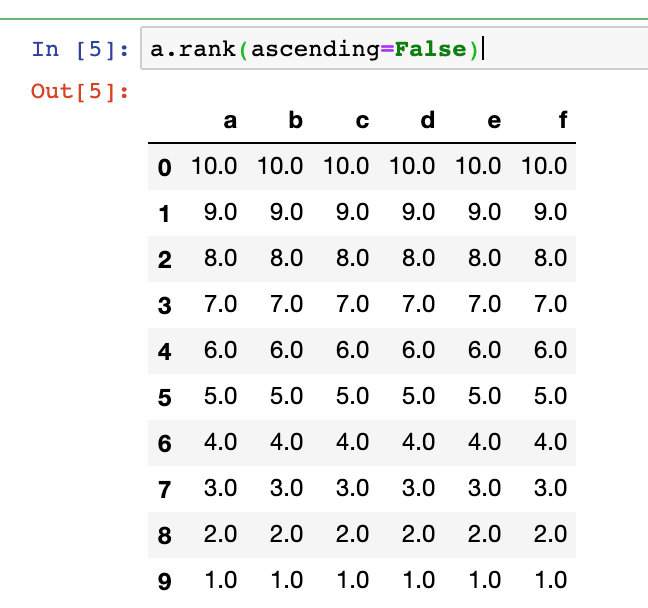 # 二十5、如何應用函數修改原dataframe-指定參數inplace pandas 中 inplace 參數在不少函數中都會有,它的做用是:是否在原對象基礎上進行修改 - inplace = True:不建立新的對象,直接對原始對象進行修改; - inplace = False:對數據進行修改,建立並返回新的對象承載其修改結果。 默認是False,即建立新的對象進行修改,原對象不變,和深複製和淺複製有些相似。
原文出處:https://www.cnblogs.com/chenqionghe/p/12197607.html